
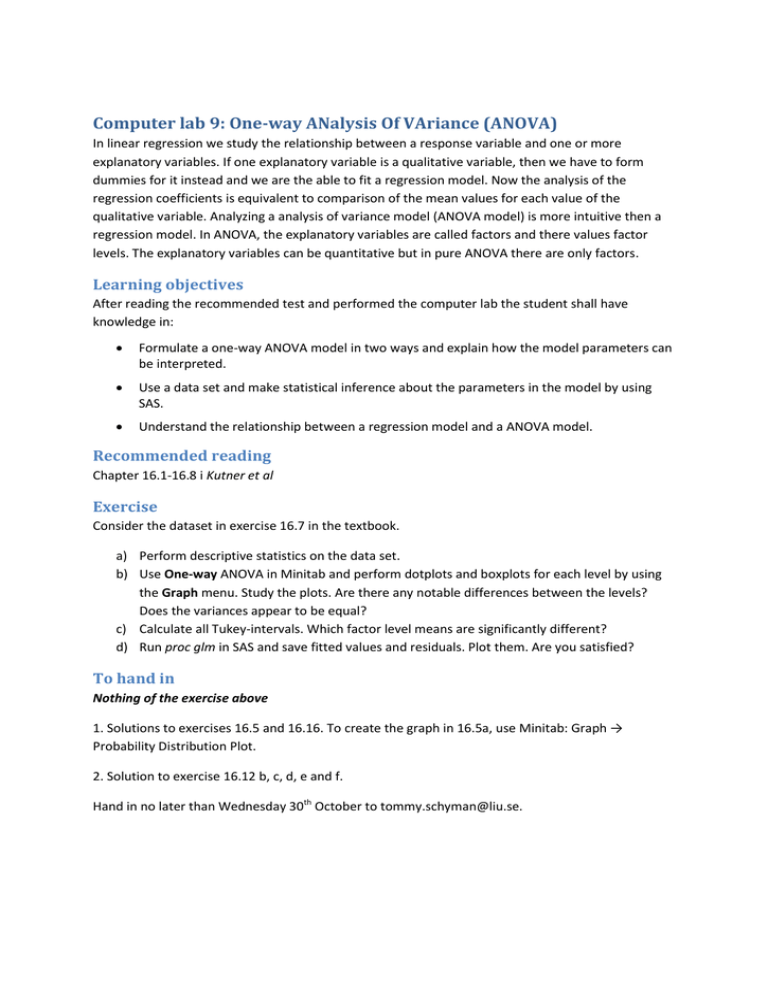
If you add a nonsignificant term, it will decrease.įinally, there is R-Sq (pred). If you add a significant term it will increase. This measure penalizes you for adding terms. If you keep adding terms to the model, it will get bigger. 60 means the model will explain 60% of the variation. It is a positive number that varies from 0 to 1. Even if all terms in your model have significant p-values, it may not be good at making predictions. Just remove terms with non significant p-values.Ģ) R-Sq shows how good the model is at explaining the variation. If the ANOVA table shows B 0 has a p-value of 0.35, and B 1 has a p-value of 0.023, you should remove the constant term from your model leaving Y = B 1X. For example, you have a simple linear regression of Y = B 0 + B 1X. :Ĭlick to expand.1) The ANOVA in Regression tests for the significance of the constant term and of the coefficients of each term in the regression model.
#MINITAB 16 ANOVA FULL#
Other times, eventhough you KNOW that the relationship exist, you can not see it because the variation of the dependent variable was too small, and the random variation of other variables came to the play, remember., as far as you can, reduce environment noise of other variables and obtain the dependent variables at least at the full lenght of the forecasting (determination) range, a little more can be of help. Sometimes, there is theory about that process that document the relationship of the variables, it can give you a start point. 1/X), if the X variation range is small enough (let's say 0.25 to 1), a lineal regression could fit with a "nice" number (r2>0.8), but if you chart the residuals, you can see a pattern on them, a pattern that will not exist if you transform the x before the regression analisys is performed (with r2=1). See Daniel and Wood for a good explaination.Ĭlick to expand.Leaving the technical aspects of regression (of course are important but.), the practical ones is to get a better estimator.Īn example of this could be seen if you obtain a lineal regression of another model, (ie. Plotting against the dependent variable allows you to see if there are patterns that would indicate a transformation. Why?Īs has been stated, least squares regressions has an assumption that the residuals are normally distributed. It is not a very good statistic as it is highly influenced by outliers and influential points.ģ) I understand what residuals are but why should one do a normality test on residuals? Also I have read that post a regression test residulas should be plotted against predicted values. It tells you how much of the variation is explained by the model. R-Sq is the coefficient of determination. There are a few statistical concepts which I encountered in my research and I am taking the liberty of asking about them as well.ġ) What is the difference between ANOVA in Regression and ANOVA in general as displayed in Minitab? In general how should one interpret ANOVA in regression?ĪNOVA is regression tells you if the model is signifcant, that is does the model explain more than just the random error of the data.Ģ) When we look at ANOVA output in Minitab we also see an R-Sq value. I have a few queries related to interpretation of certain terms in Minitab related to Regression (GLM) and ANOVA.
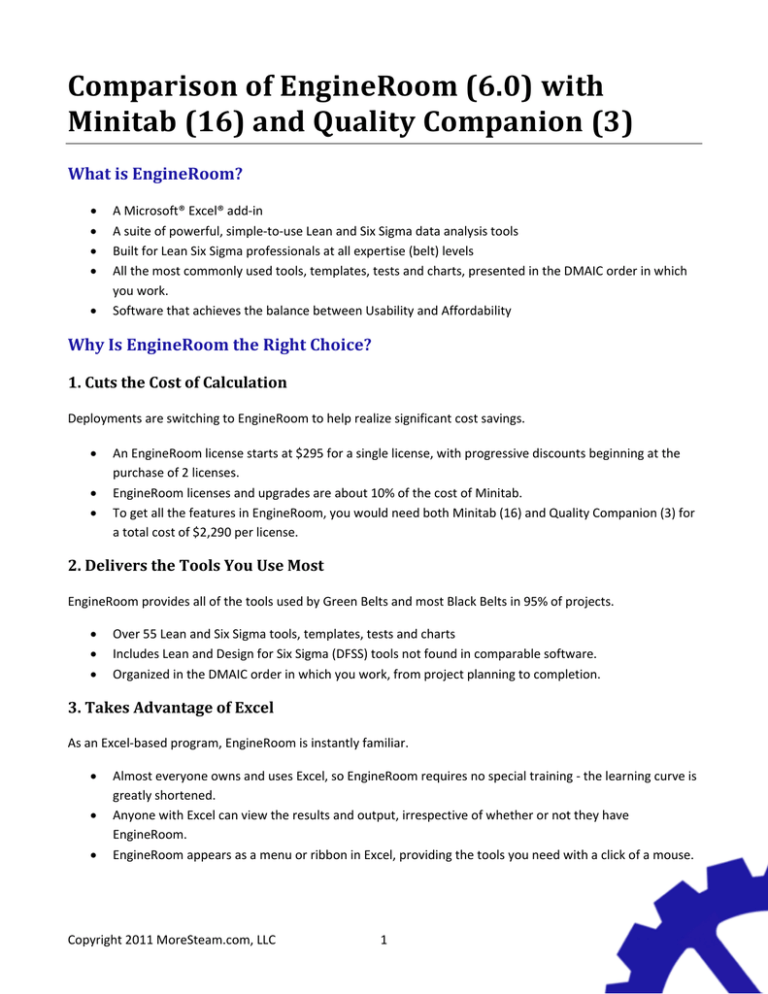
The theory of regression (using the least-squares fit) assumes that you are able to explain all of the variation in the Y data, with the exception of a set of residual data, which are independent from each other, and randomly distributed as a Normal random variable with mean zero, and some variance (which is calculated by the ANOVA). ANOVA helps me to show how much of the variation is within the fit versus left over in the residuals. The hope is that I have explained the variation in the Y data through the regression, and only have left a set of residual data. When I do a regression (be it linear or non-linear, single or multiple) I am trying to "explain" some component of variation in the Y data based upon X1, X2.
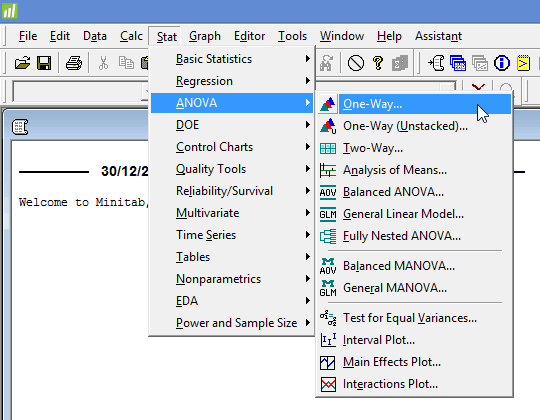
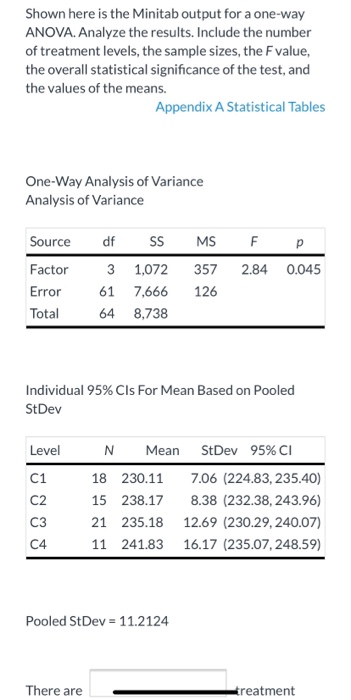
There is a residual term which expresses the difference between the variance within each data set and compared to the variance between each data set. Yes, at first though you think about comparing two or more data sets and trying to conclude if they are the same or different, and ANOVA does this through comparing their variances. ANOVA is literally an analysis of variance.
